图书介绍
系统辨识 使用者的理论 英文版PDF|Epub|txt|kindle电子书版本下载
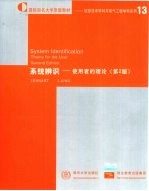
- Lennart Ljung等著 著
- 出版社: 北京:清华大学出版社
- ISBN:7302051437
- 出版时间:2002
- 标注页数:613页
- 文件大小:21MB
- 文件页数:635页
- 主题词:暂缺
PDF下载
下载说明
系统辨识 使用者的理论 英文版PDF格式电子书版下载
下载的文件为RAR压缩包。需要使用解压软件进行解压得到PDF格式图书。建议使用BT下载工具Free Download Manager进行下载,简称FDM(免费,没有广告,支持多平台)。本站资源全部打包为BT种子。所以需要使用专业的BT下载软件进行下载。如BitComet qBittorrent uTorrent等BT下载工具。迅雷目前由于本站不是热门资源。不推荐使用!后期资源热门了。安装了迅雷也可以迅雷进行下载!
(文件页数 要大于 标注页数,上中下等多册电子书除外)
注意:本站所有压缩包均有解压码: 点击下载压缩包解压工具
图书目录
(1 Introduction1
1.1 Dynamic Systems1
1.2 Models6
1.3 An Archetypical Problem-ARX Models and the Linear Least Squares Method8
1.4 The System Identification Procedure13
1.5 Organization of the Book14
1.6 Bibliography16
(2 Time-Invariant Linear Systems18
2.1 Impulse Responses, Disturbances, and Transfer Functions18
2.2 Frequency-Domain Expressions28
2.3 Signal Spectra33
2.4 Single Realization Behavior and Ergodicity Results(*)42
2.5 Multivariable Systems(*)44
2.6 Summary45
2.7 Bibliography46
2.8 Problems47
Appendix 2A: Proof of Theorem 2.252
Appendix 2B: Proof of Theorem 2.355
Appendix 2C: Covariance Formulas61
3.1 Simulation63
(3 Simulation and Predition63
3.2 Prediction64
3.3 Observers72
3.4 Summary75
3.5 Bibliography75
3.6 Problems76
(4 Models of Linear Time-Invariant Systems79
4.1 Linear Models and Sets of Linear Models79
4.2 A Family of Transfer-Function Models81
4.3 State-Space Models93
4.4 Distributed Parameter Models(*)103
4.5 Model Sets, Model Structures, and Identifiability: Some Formal Aspects(*)105
4.6 Identifiability of Some Model Structures114
4.7 Summary118
4.8 Bibliography119
4.9 Problems121
Appendix 4A: Identifiability of Black-Box Multivariable Model Structures128
(5 Models for Time-varying and Nonlinear Systems140
5.1 Linear Time-Varying Models140
5.2 Models with Nonlinearities143
5.3 Nonlinear State-Space Models146
5.4 Nonlinear Black-Box Models: Basic Principles148
5.5 Nonlinear Black-Box Models: Neural Networks, Wavelets and Classical Models154
5.6 Fuzzy Models156
5.7 Formal Characterization of Models(*)161
5.8 Summary164
5.9 Bibliography165
5.10 Problems165
(6 Nonparametric Time-and Frequency-Domain Methods168
6.1 Transient-Response Analysis and Correlation Analysis168
6.2 Frequency-Response Analysis170
6.3 Fourier Analysis173
6.4 Spectral Analysis178
6.5 Estimating the Disturbance Spectrum(*)187
6.6 Summary189
6.7 Bibliography190
6.8 Problems191
Appendix 6A: Derivation of the Asymptotic Properties of the Spectral Analysis Estimate194
(7 Parameter Estimation Methods197
7.1 Guiding Principles Behind Parameter Estimation Methods197
7.2 Minimizing Prediction Errors199
7.3 Linear Regressions and the Least-Squares Method203
7.4 A Statistical Framework for Parameter Estimation and the Maximum Likelihood Method212
7.5 Correlating Prediction Errors with Past Data222
7.6 Instrumental-Variable Methods224
7.7 Using Frequency Domain Data to Fit Linear Models(*)227
7.8 Summary233
7.9 Bibliography234
7.10 Problems236
Appendix 7A: Proof of the Cramér-Rao Inequality245
(8 Convergence and Consistency247
8.1 Introduction247
8.2 Conditions on the Data Set249
8.3 Prediction-Error Approach253
8.4 Consistency and Identifiability258
8.5 Linear Time-Invariant Models:A Frequency-Domain Description of the Limit Model263
8.6 The Correlation Approach269
8.7 Summary273
8.8 Bibliography274
8.9 Problems275
(9 Asymptotic Distribution of Parameter Estimates280
9.1 Introduction280
9.2 The Prediction-Error Approach: Basic Theorem281
9.3 Expressions for the Asymptotic Variance283
9.4 Frequency-Domain Expressions for the Asymptotic Variance290
9.5 The Correlation Approach296
9.6 Use and Relevance of Asymptotic Variance Expressions302
9.7 Summary304
9.8 Bibliography305
9.9 Problems305
Appendix 9A: Proof of Theorem 9.1309
Appendix 9B: The Asymptotic Parameter Variance313
(10 Computing the Estimate317
10.1 Linear Regressions and Least Squares317
10.2 Numerical Solution by Iterative Search Methods326
10.3 Computing Gradients329
10.4 Two-Stage and Multistage Methods333
10.5 Local Solutions and Initial Values338
10.6 Subspace Methods for Estimating State Space Models340
10.7 Summary351
10.8 Bibliography352
10.9 Problems353
11 Recursive Estimation Methods361
11.1 Introduction361
11.2 The Recursive Least-Squares Algorithm363
11.3 The Recursive IV Method369
11.4 Recursive Prediction-Error Methods370
11.5 Recursive Pseudolinear Regressions374
11.6 The Choice of Updating Step376
11.7 Implementation382
11.8 Summary386
11.9 Bibliography387
11.10 Problems388
Appendix 11A: Techniques for Asymptotic Analysis of Recursive Algorithms389
11A Problems398
(12 Options and Objectives399
12.1 Options399
12.2 Objectives400
12.3 Bias and Variance404
12.4 Summary406
12.5 Bibliography406
12.6 Problems406
(13 Experiment Design408
13.1 Some General Considerations408
13.2 Informative Experiments411
13.3 Input Design for Open Loop Experiments415
13.4 Identification in Closed Loop:Identifiability428
13.5 Approaches to Closed Loop Identification434
13.6 Optimal Experiment Design for High-Order Black-Box Models441
13.7 Choice of Sampling Interval and Presampling Filters444
13.8 Summary452
13.9 Bibliography453
13.10 Problems454
(14 Preprocessing Data458
14.1 Drifts and Detrending458
14.2 Outliers and Missing Data461
14.3 Selecting Segments of Data and Merging Experiments464
14.4 Prefiltering466
14.5 Formal Design of Prefiltering and Input Properties470
14.6 Summary474
14.8 Problems475
14.7 Bibliography475
(15 Choice of Identification Criterion477
15.1 General Aspects477
15.2 Choice of Norm: Robustness479
15.3 Variance-Optimal Instruments485
15.4 Summary488
15.5 Bibliography489
15.6 Problems490
(16 Model Structure Selection and Model Validation491
16.1 General Aspects of the Choice of Model Structure491
16.2 A Priori Considerations493
16.3 Model Structure Selection Based on Preliminary Data Analysis495
16.4 Comparing Model Structures498
16.5 Model Validation509
16.6 Residual Analysis511
16.7 Summary516
16.8 Bibliography517
16.9 Problems518
(17 System Identification in Practice520
17.1 The Tool:Interactive Software520
17.2 The Practical Side of System Identification522
17.3 Some Applications525
17.4 What Does System Identification Have To Offer?536
(AppendixⅠSome Concepts From Probability Theory539
( AppendixⅡ Some Statistical Techniques for Linear Regressions543
Ⅱ.1 Linear Regressions and the Least Squares Estimate543
Ⅱ.2 Statistical Properties of the Least-Squares Estimate551
Ⅱ.3 Some Further Topics in Least-Squares Estimation559
Ⅱ.4 Problems564
References565
Subject Index596
Reference Index603